generated from xuyuqing/ailab
add model
This commit is contained in:
parent
a79c56c977
commit
478de9d646
|
@ -0,0 +1 @@
|
|||
*.bin filter=lfs diff=lfs merge=lfs -text
|
Binary file not shown.
Binary file not shown.
140
README.md
140
README.md
|
@ -0,0 +1,140 @@
|
|||
---
|
||||
language:
|
||||
- zh
|
||||
- en
|
||||
pipeline_tag: text-generation
|
||||
inference: false
|
||||
---
|
||||
# Baichuan-13B-Base
|
||||
|
||||
<!-- Provide a quick summary of what the model is/does. -->
|
||||
|
||||
## 介绍
|
||||
Baichuan-13B-Base为Baichuan-13B系列模型中的预训练版本,经过对齐后的模型可见[Baichuan-13B-Chat](https://huggingface.co/baichuan-inc/Baichuan-13B-Chat)。
|
||||
|
||||
[Baichuan-13B](https://github.com/baichuan-inc/Baichuan-13B) 是由百川智能继 [Baichuan-7B](https://github.com/baichuan-inc/baichuan-7B) 之后开发的包含 130 亿参数的开源可商用的大规模语言模型,在权威的中文和英文 benchmark 上均取得同尺寸最好的效果。本次发布包含有预训练 ([Baichuan-13B-Base](https://huggingface.co/baichuan-inc/Baichuan-13B-Base)) 和对齐 ([Baichuan-13B-Chat](https://huggingface.co/baichuan-inc/Baichuan-13B-Chat)) 两个版本。Baichuan-13B 有如下几个特点:
|
||||
|
||||
1. **更大尺寸、更多数据**:Baichuan-13B 在 [Baichuan-7B](https://github.com/baichuan-inc/baichuan-7B) 的基础上进一步扩大参数量到 130 亿,并且在高质量的语料上训练了 1.4 万亿 tokens,超过 LLaMA-13B 40%,是当前开源 13B 尺寸下训练数据量最多的模型。支持中英双语,使用 ALiBi 位置编码,上下文窗口长度为 4096。
|
||||
2. **同时开源预训练和对齐模型**:预训练模型是适用开发者的“基座”,而广大普通用户对有对话功能的对齐模型具有更强的需求。因此本次开源我们同时发布了对齐模型(Baichuan-13B-Chat),具有很强的对话能力,开箱即用,几行代码即可简单的部署。
|
||||
3. **更高效的推理**:为了支持更广大用户的使用,我们本次同时开源了 int8 和 int4 的量化版本,相对非量化版本在几乎没有效果损失的情况下大大降低了部署的机器资源门槛,可以部署在如 Nvidia 3090 这样的消费级显卡上。
|
||||
4. **开源免费可商用**:Baichuan-13B 不仅对学术研究完全开放,开发者也仅需邮件申请并获得官方商用许可后,即可以免费商用。
|
||||
5.
|
||||
Baichuan-13B-Base is the pre-training version in the Baichuan-13B series of models, and the aligned model can be found at [Baichuan-13B-Chat](https://huggingface.co/baichuan-inc/Baichuan-13B-Chat).
|
||||
|
||||
[Baichuan-13B](https://github.com/baichuan-inc/Baichuan-13B) is an open-source, commercially usable large-scale language model developed by Baichuan Intelligence, following [Baichuan-7B](https://github.com/baichuan-inc/baichuan-7B). With 13 billion parameters, it achieves the best performance in standard Chinese and English benchmarks among models of its size. This release includes two versions: pre-training (Baichuan-13B-Base) and alignment (Baichuan-13B-Chat). Baichuan-13B has the following features:
|
||||
|
||||
1. **Larger size, more data**: Baichuan-13B further expands the parameter volume to 13 billion based on [Baichuan-7B](https://github.com/baichuan-inc/baichuan-7B), and has trained 1.4 trillion tokens on high-quality corpora, exceeding LLaMA-13B by 40%. It is currently the model with the most training data in the open-source 13B size. It supports both Chinese and English, uses ALiBi position encoding, and has a context window length of 4096.
|
||||
2. **Open-source pre-training and alignment models simultaneously**: The pre-training model is a "base" suitable for developers, while the general public has a stronger demand for alignment models with dialogue capabilities. Therefore, in this open-source release, we also released the alignment model (Baichuan-13B-Chat), which has strong dialogue capabilities and is ready to use. It can be easily deployed with just a few lines of code.
|
||||
3. **More efficient inference**: To support a wider range of users, we have open-sourced the INT8 and INT4 quantized versions. The model can be conveniently deployed on consumer GPUs like the Nvidia 3090 with almost no performance loss.
|
||||
4. **Open-source, free, and commercially usable**: Baichuan-13B is not only fully open to academic research, but developers can also use it for free commercially after applying for and receiving official commercial permission via email.
|
||||
|
||||
|
||||
## 模型详情
|
||||
|
||||
### 模型描述
|
||||
|
||||
<!-- Provide a longer summary of what this model is. -->
|
||||
|
||||
- **Developed by:** 百川智能(Baichuan Intelligent Technology)
|
||||
- **Email**: opensource@baichuan-inc.com
|
||||
- **Language(s) (NLP):** Chinese/English
|
||||
- **License:** 【Community License for Baichuan-13B Model】([ZH](Baichuan-13B%20%E6%A8%A1%E5%9E%8B%E5%95%86%E7%94%A8%E8%AE%B8%E5%8F%AF%E5%8D%8F%E8%AE%AE.pdf)|
|
||||
[EN](Community%20License%20for%20Baichuan-13B%20Model.pdf))
|
||||
|
||||
**商业用途还需遵循(For commercial use additional):** 请通过 [Email](mailto:opensource@baichuan-inc.com) 联系申请书面授权。(Contact us via [Email](mailto:opensource@baichuan-inc.com) above to apply for written authorization.)
|
||||
|
||||
### 模型结构
|
||||
|
||||
<!-- Provide the basic links for the model. -->
|
||||
|
||||
整体模型基于Baichuan-7B,为了获得更好的推理性能,Baichuan-13B 使用了 ALiBi 线性偏置技术,相对于 Rotary Embedding 计算量更小,对推理性能有显著提升;与标准的 LLaMA-13B 相比,生成 2000 个 tokens 的平均推理速度 (tokens/s),实测提升 31.6%:
|
||||
|
||||
| Model | tokens/s |
|
||||
|-------------|----------|
|
||||
| LLaMA-13B | 19.4 |
|
||||
| Baichuan-13B| 25.4 |
|
||||
|
||||
具体参数和见下表
|
||||
| 模型名称 | 隐含层维度 | 层数 | 头数 |词表大小 | 总参数量 | 训练数据(tokens) | 位置编码 | 最大长度 |
|
||||
|-------------------------|-------|------------|------------|-----------------|--------|--------|----------------|---------|
|
||||
| Baichuan-7B | 4,096 | 32 | 32 | 64,000 | 7,000,559,616 | 1.2万亿 | [RoPE](https://arxiv.org/abs/2104.09864) | 4,096 |
|
||||
| Baichuan-13B | 5,120 | 40 | 40 | 64,000 | 13,264,901,120 | 1.4万亿 | [ALiBi](https://arxiv.org/abs/2108.12409) | 4,096
|
||||
|
||||
The overall model is based on Baichuan-7B. In order to achieve better inference performance, Baichuan-13B uses ALiBi linear bias technology, which has a smaller computational load compared to Rotary Embedding, and significantly improves inference performance. Compared with the standard LLaMA-13B, the average inference speed (tokens/s) for generating 2000 tokens has been tested to increase by 31.6%:
|
||||
|
||||
| Model | tokens/s |
|
||||
|-------------|----------|
|
||||
| LLaMA-13B | 19.4 |
|
||||
| Baichuan-13B| 25.4 |
|
||||
|
||||
The specific parameters are as follows:
|
||||
| Model Name | Hidden Size | Num Layers | Num Attention Heads |Vocab Size | Total Params | Training Dats(tokens) | Position Embedding | Max Length |
|
||||
|-------------------------|-------|------------|------------|-----------------|--------|--------|----------------|---------|
|
||||
| Baichuan-7B | 4,096 | 32 | 32 | 64,000 | 7,000,559,616 | 1.2万亿 | [RoPE](https://arxiv.org/abs/2104.09864) | 4,096 |
|
||||
| Baichuan-13B | 5,120 | 40 | 40 | 64,000 | 13,264,901,120 | 1.4万亿 | [ALiBi](https://arxiv.org/abs/2108.12409) | 4,096
|
||||
|
||||
### 免责声明
|
||||
|
||||
我们在此声明,我们的开发团队并未基于 Baichuan-13B 模型开发任何应用,无论是在 iOS、Android、网页或任何其他平台。我们强烈呼吁所有使用者,不要利用 Baichuan-13B 模型进行任何危害国家社会安全或违法的活动。另外,我们也要求使用者不要将 Baichuan-13B 模型用于未经适当安全审查和备案的互联网服务。我们希望所有的使用者都能遵守这个原则,确保科技的发展能在规范和合法的环境下进行。
|
||||
|
||||
我们已经尽我们所能,来确保模型训练过程中使用的数据的合规性。然而,尽管我们已经做出了巨大的努力,但由于模型和数据的复杂性,仍有可能存在一些无法预见的问题。因此,如果由于使用 Baichuan-13B 开源模型而导致的任何问题,包括但不限于数据安全问题、公共舆论风险,或模型被误导、滥用、传播或不当利用所带来的任何风险和问题,我们将不承担任何责任。
|
||||
|
||||
We hereby declare that our development team has not developed any applications based on the Baichuan-13B model, whether on iOS, Android, the web, or any other platform. We strongly urge all users not to use the Baichuan-13B model for any activities that harm national social security or are illegal. In addition, we also ask users not to use the Baichuan-13B model for internet services that have not undergone appropriate security review and filing. We hope that all users will adhere to this principle to ensure that technological development takes place in a regulated and legal environment.
|
||||
|
||||
We have done our utmost to ensure the compliance of the data used in the model training process. However, despite our great efforts, due to the complexity of the model and data, there may still be some unforeseen issues. Therefore, we will not take any responsibility for any issues arising from the use of the Baichuan-13B open-source model, including but not limited to data security issues, public opinion risks, or any risks and problems arising from the model being misled, misused, disseminated, or improperly exploited.
|
||||
|
||||
## 训练详情
|
||||
|
||||
训练具体设置参见[Baichuan-13B](https://github.com/baichuan-inc/Baichuan-13B)。
|
||||
|
||||
For specific training settings, please refer to [Baichuan-13B](https://github.com/baichuan-inc/Baichuan-13B).
|
||||
|
||||
## 测评结果
|
||||
|
||||
### [C-Eval](https://cevalbenchmark.com/index.html#home)
|
||||
|
||||
| Model 5-shot | STEM | Social Sciences | Humanities | Others | Average |
|
||||
|-------------------------|:-----:|:---------------:|:----------:|:------:|:-------:|
|
||||
| Baichuan-7B | 38.2 | 52.0 | 46.2 | 39.3 | 42.8 |
|
||||
| Chinese-Alpaca-Plus-13B | 35.2 | 45.6 | 40.0 | 38.2 | 38.8 |
|
||||
| Vicuna-13B | 30.5 | 38.2 | 32.5 | 32.5 | 32.8 |
|
||||
| Chinese-LLaMA-Plus-13B | 30.3 | 38.0 | 32.9 | 29.1 | 32.1 |
|
||||
| Ziya-LLaMA-13B-Pretrain | 27.6 | 34.4 | 32.0 | 28.6 | 30.0 |
|
||||
| LLaMA-13B | 27.0 | 33.6 | 27.7 | 27.6 | 28.5 |
|
||||
| moss-moon-003-base (16B)| 27.0 | 29.1 | 27.2 | 26.9 | 27.4 |
|
||||
| **Baichuan-13B-Base** | **45.9** | **63.5** | **57.2** | **49.3** | **52.4** |
|
||||
| **Baichuan-13B-Chat** | **43.7** | **64.6** | **56.2** | **49.2** | **51.5** |
|
||||
|
||||
|
||||
### [MMLU](https://arxiv.org/abs/2009.03300)
|
||||
|
||||
| Model 5-shot | STEM | Social Sciences | Humanities | Others | Average |
|
||||
|-------------------------|:-----:|:---------------:|:----------:|:------:|:-------:|
|
||||
| Vicuna-13B | 40.4 | 60.5 | 49.5 | 58.4 | 52.0 |
|
||||
| LLaMA-13B | 36.1 | 53.0 | 44.0 | 52.8 | 46.3 |
|
||||
| Chinese-Alpaca-Plus-13B | 36.9 | 48.9 | 40.5 | 50.5 | 43.9 |
|
||||
| Ziya-LLaMA-13B-Pretrain | 35.6 | 47.6 | 40.1 | 49.4 | 42.9 |
|
||||
| Baichuan-7B | 35.6 | 48.9 | 38.4 | 48.1 | 42.3 |
|
||||
| Chinese-LLaMA-Plus-13B | 33.1 | 42.8 | 37.0 | 44.6 | 39.2 |
|
||||
| moss-moon-003-base (16B)| 22.4 | 22.8 | 24.2 | 24.4 | 23.6 |
|
||||
| **Baichuan-13B-Base** | **41.6** | **60.9** | **47.4** | **58.5** | **51.6** |
|
||||
| **Baichuan-13B-Chat** | **40.9** | **60.9** | **48.8** | **59.0** | **52.1** |
|
||||
> 说明:我们采用了 MMLU 官方的[评测方案](https://github.com/hendrycks/test)。
|
||||
|
||||
### [CMMLU](https://github.com/haonan-li/CMMLU)
|
||||
|
||||
| Model 5-shot | STEM | Humanities | Social Sciences | Others | China Specific | Average |
|
||||
|-------------------------|:-----:|:----------:|:---------------:|:------:|:--------------:|:-------:|
|
||||
| Baichuan-7B | 34.4 | 47.5 | 47.6 | 46.6 | 44.3 | 44.0 |
|
||||
| Vicuna-13B | 31.8 | 36.2 | 37.6 | 39.5 | 34.3 | 36.3 |
|
||||
| Chinese-Alpaca-Plus-13B | 29.8 | 33.4 | 33.2 | 37.9 | 32.1 | 33.4 |
|
||||
| Chinese-LLaMA-Plus-13B | 28.1 | 33.1 | 35.4 | 35.1 | 33.5 | 33.0 |
|
||||
| Ziya-LLaMA-13B-Pretrain | 29.0 | 30.7 | 33.8 | 34.4 | 31.9 | 32.1 |
|
||||
| LLaMA-13B | 29.2 | 30.8 | 31.6 | 33.0 | 30.5 | 31.2 |
|
||||
| moss-moon-003-base (16B)| 27.2 | 30.4 | 28.8 | 32.6 | 28.7 | 29.6 |
|
||||
| **Baichuan-13B-Base** | **41.7** | **61.1** | **59.8** | **59.0** | **56.4** | **55.3** |
|
||||
| **Baichuan-13B-Chat** | **42.8** | **62.6** | **59.7** | **59.0** | **56.1** | **55.8** |
|
||||
> 说明:CMMLU 是一个综合性的中文评估基准,专门用于评估语言模型在中文语境下的知识和推理能力。我们采用了其官方的[评测方案](https://github.com/haonan-li/CMMLU)。
|
||||
|
||||
## 微信群组
|
||||
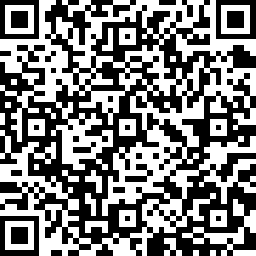
|
|
@ -0,0 +1,28 @@
|
|||
{
|
||||
"_from_model_config": true,
|
||||
"architectures": [
|
||||
"BaichuanForCausalLM"
|
||||
],
|
||||
"auto_map": {
|
||||
"AutoConfig": "configuration_baichuan.BaichuanConfig",
|
||||
"AutoModelForCausalLM": "modeling_baichuan.BaichuanForCausalLM"
|
||||
},
|
||||
"bos_token_id": 1,
|
||||
"eos_token_id": 2,
|
||||
"gradient_checkpointing": false,
|
||||
"hidden_act": "silu",
|
||||
"hidden_size": 5120,
|
||||
"initializer_range": 0.02,
|
||||
"intermediate_size": 13696,
|
||||
"model_max_length": 4096,
|
||||
"model_type": "baichuan",
|
||||
"num_attention_heads": 40,
|
||||
"num_hidden_layers": 40,
|
||||
"pad_token_id": 0,
|
||||
"rms_norm_eps": 1e-06,
|
||||
"tie_word_embeddings": false,
|
||||
"torch_dtype": "bfloat16",
|
||||
"transformers_version": "4.29.2",
|
||||
"use_cache": true,
|
||||
"vocab_size": 64000
|
||||
}
|
|
@ -0,0 +1,46 @@
|
|||
# Copyright (c) 2023, Baichuan Intelligent Technology. All rights reserved.
|
||||
|
||||
from transformers.configuration_utils import PretrainedConfig
|
||||
|
||||
class BaichuanConfig(PretrainedConfig):
|
||||
model_type = "baichuan"
|
||||
keys_to_ignore_at_inference = ["past_key_values"]
|
||||
|
||||
def __init__(
|
||||
self,
|
||||
vocab_size=64000,
|
||||
hidden_size=5120,
|
||||
intermediate_size=13696,
|
||||
num_hidden_layers=40,
|
||||
num_attention_heads=40,
|
||||
hidden_act="silu",
|
||||
model_max_length=4096,
|
||||
initializer_range=0.02,
|
||||
rms_norm_eps=1e-6,
|
||||
use_cache=True,
|
||||
pad_token_id=0,
|
||||
bos_token_id=1,
|
||||
eos_token_id=2,
|
||||
tie_word_embeddings=False,
|
||||
gradient_checkpointing=False,
|
||||
**kwargs,
|
||||
):
|
||||
self.vocab_size = vocab_size
|
||||
self.model_max_length = model_max_length
|
||||
self.hidden_size = hidden_size
|
||||
self.intermediate_size = intermediate_size
|
||||
self.num_hidden_layers = num_hidden_layers
|
||||
self.num_attention_heads = num_attention_heads
|
||||
self.hidden_act = hidden_act
|
||||
self.initializer_range = initializer_range
|
||||
self.rms_norm_eps = rms_norm_eps
|
||||
self.use_cache = use_cache
|
||||
self.gradient_checkpointing = gradient_checkpointing,
|
||||
super().__init__(
|
||||
pad_token_id=pad_token_id,
|
||||
bos_token_id=bos_token_id,
|
||||
eos_token_id=eos_token_id,
|
||||
tie_word_embeddings=tie_word_embeddings,
|
||||
**kwargs,
|
||||
)
|
||||
|
|
@ -0,0 +1,7 @@
|
|||
{
|
||||
"_from_model_config": true,
|
||||
"bos_token_id": 1,
|
||||
"eos_token_id": 2,
|
||||
"pad_token_id": 0,
|
||||
"transformers_version": "4.29.2"
|
||||
}
|
|
@ -0,0 +1,536 @@
|
|||
# Copyright (c) 2023, Baichuan Intelligent Technology. All rights reserved.
|
||||
|
||||
import math
|
||||
from typing import List, Optional, Tuple, Union
|
||||
|
||||
import torch
|
||||
import torch.utils.checkpoint
|
||||
from torch.nn import CrossEntropyLoss
|
||||
from transformers import PreTrainedModel
|
||||
from transformers.activations import ACT2FN
|
||||
from transformers.modeling_outputs import BaseModelOutputWithPast, CausalLMOutputWithPast
|
||||
from transformers.utils import logging
|
||||
from transformers.generation.utils import GenerationConfig
|
||||
|
||||
from .configuration_baichuan import BaichuanConfig
|
||||
|
||||
logger = logging.get_logger(__name__)
|
||||
|
||||
def _get_interleave(n):
|
||||
def _get_interleave_power_of_2(n):
|
||||
start = (2 ** (-2 ** -(math.log2(n) - 3)))
|
||||
ratio = start
|
||||
return [start * ratio ** i for i in range(n)]
|
||||
|
||||
if math.log2(n).is_integer():
|
||||
return _get_interleave_power_of_2(n)
|
||||
else:
|
||||
closest_power_of_2 = 2 ** math.floor(math.log2(n))
|
||||
return _get_interleave_power_of_2(closest_power_of_2) + \
|
||||
_get_interleave(2 * closest_power_of_2)[0::2][:n - closest_power_of_2]
|
||||
|
||||
def _fill_with_neg_inf(t):
|
||||
"""FP16-compatible function that fills a tensor with -inf."""
|
||||
return t.float().fill_(float("-inf")).type_as(t)
|
||||
|
||||
def _gen_alibi_mask(n_head, max_pos):
|
||||
slopes = torch.Tensor(_get_interleave(n_head))
|
||||
alibi = slopes.unsqueeze(1).unsqueeze(1) * torch.arange(max_pos).unsqueeze(0).unsqueeze(0).expand(
|
||||
n_head, -1, -1)
|
||||
alibi = alibi.view(n_head, 1, max_pos)
|
||||
alibi_mask = torch.triu(
|
||||
_fill_with_neg_inf(torch.zeros([max_pos, max_pos])), 1
|
||||
)
|
||||
alibi_mask = alibi_mask.unsqueeze(0) + alibi
|
||||
return alibi_mask
|
||||
|
||||
|
||||
class RMSNorm(torch.nn.Module):
|
||||
def __init__(self, hidden_size, epsilon=1e-6):
|
||||
super().__init__()
|
||||
self.weight = torch.nn.Parameter(torch.empty(hidden_size))
|
||||
self.epsilon = epsilon
|
||||
|
||||
def forward(self, hidden_states):
|
||||
variance = hidden_states.to(torch.float32).pow(2).mean(-1, keepdim=True)
|
||||
hidden_states = hidden_states * torch.rsqrt(variance + self.epsilon)
|
||||
|
||||
# convert into half-precision
|
||||
if self.weight.dtype in [torch.float16, torch.bfloat16]:
|
||||
hidden_states = hidden_states.to(self.weight.dtype)
|
||||
|
||||
return self.weight * hidden_states
|
||||
|
||||
|
||||
class MLP(torch.nn.Module):
|
||||
def __init__(
|
||||
self,
|
||||
hidden_size: int,
|
||||
intermediate_size: int,
|
||||
hidden_act: str,
|
||||
):
|
||||
super().__init__()
|
||||
self.gate_proj = torch.nn.Linear(hidden_size, intermediate_size, bias=False)
|
||||
self.down_proj = torch.nn.Linear(intermediate_size, hidden_size, bias=False)
|
||||
self.up_proj = torch.nn.Linear(hidden_size, intermediate_size, bias=False)
|
||||
self.act_fn = ACT2FN[hidden_act]
|
||||
|
||||
def forward(self, x):
|
||||
return self.down_proj(self.act_fn(self.gate_proj(x)) * self.up_proj(x))
|
||||
|
||||
|
||||
class BaichuanAttention(torch.nn.Module):
|
||||
|
||||
def __init__(self, config: BaichuanConfig):
|
||||
super().__init__()
|
||||
self.config = config
|
||||
self.hidden_size = config.hidden_size
|
||||
self.num_heads = config.num_attention_heads
|
||||
self.head_dim = self.hidden_size // self.num_heads
|
||||
self.max_position_embeddings = config.model_max_length
|
||||
|
||||
if (self.head_dim * self.num_heads) != self.hidden_size:
|
||||
raise ValueError(
|
||||
f"hidden_size {self.hidden_size} is not divisible by num_heads {self.num_heads}"
|
||||
)
|
||||
self.W_pack = torch.nn.Linear(self.hidden_size, 3 * self.hidden_size, bias=False)
|
||||
self.o_proj = torch.nn.Linear(self.num_heads * self.head_dim, self.hidden_size, bias=False)
|
||||
|
||||
def _shape(self, tensor: torch.Tensor, seq_len: int, bsz: int):
|
||||
return tensor.view(bsz, seq_len, self.num_heads, self.head_dim).transpose(1, 2).contiguous()
|
||||
|
||||
def forward(
|
||||
self,
|
||||
hidden_states: torch.Tensor,
|
||||
attention_mask: Optional[torch.Tensor] = None,
|
||||
past_key_value: Optional[Tuple[torch.Tensor]] = None,
|
||||
output_attentions: bool = False,
|
||||
use_cache: bool = False,
|
||||
) -> Tuple[torch.Tensor, Optional[torch.Tensor], Optional[Tuple[torch.Tensor]]]:
|
||||
|
||||
bsz, q_len, _ = hidden_states.size()
|
||||
|
||||
proj = self.W_pack(hidden_states)
|
||||
proj = proj.unflatten(-1, (3, self.hidden_size)).unsqueeze(0).transpose(0, -2).squeeze(-2)
|
||||
query_states = proj[0].view(bsz, q_len, self.num_heads, self.head_dim).transpose(1, 2)
|
||||
key_states = proj[1].view(bsz, q_len, self.num_heads, self.head_dim).transpose(1, 2)
|
||||
value_states = proj[2].view(bsz, q_len, self.num_heads, self.head_dim).transpose(1, 2)
|
||||
|
||||
kv_seq_len = key_states.shape[-2]
|
||||
if past_key_value is not None:
|
||||
kv_seq_len += past_key_value[0].shape[-2]
|
||||
|
||||
if past_key_value is not None:
|
||||
# reuse k, v, self_attention
|
||||
key_states = torch.cat([past_key_value[0], key_states], dim=2)
|
||||
value_states = torch.cat([past_key_value[1], value_states], dim=2)
|
||||
|
||||
past_key_value = (key_states, value_states) if use_cache else None
|
||||
|
||||
attn_weights = torch.matmul(query_states, key_states.transpose(2, 3)) / math.sqrt(self.head_dim)
|
||||
|
||||
if attention_mask is not None:
|
||||
if attn_weights.size(-2) == 1:
|
||||
attention_mask = attention_mask[:, -1:, :]
|
||||
attn_weights = attn_weights + attention_mask.unsqueeze(0)
|
||||
attn_weights = torch.max(attn_weights, torch.tensor(torch.finfo(attn_weights.dtype).min))
|
||||
|
||||
attn_weights = torch.nn.functional.softmax(attn_weights, dim=-1)
|
||||
attn_output = torch.matmul(attn_weights, value_states)
|
||||
|
||||
attn_output = attn_output.transpose(1, 2)
|
||||
attn_output = attn_output.reshape(bsz, q_len, self.hidden_size)
|
||||
attn_output = self.o_proj(attn_output)
|
||||
|
||||
if not output_attentions:
|
||||
attn_weights = None
|
||||
|
||||
return attn_output, attn_weights, past_key_value
|
||||
|
||||
|
||||
class BaichuanLayer(torch.nn.Module):
|
||||
def __init__(self, config: BaichuanConfig):
|
||||
super().__init__()
|
||||
self.hidden_size = config.hidden_size
|
||||
self.self_attn = BaichuanAttention(config=config)
|
||||
self.mlp = MLP(
|
||||
hidden_size=self.hidden_size,
|
||||
intermediate_size=config.intermediate_size,
|
||||
hidden_act=config.hidden_act,
|
||||
)
|
||||
self.input_layernorm = RMSNorm(config.hidden_size, epsilon=config.rms_norm_eps)
|
||||
self.post_attention_layernorm = RMSNorm(config.hidden_size, epsilon=config.rms_norm_eps)
|
||||
|
||||
def forward(
|
||||
self,
|
||||
hidden_states: torch.Tensor,
|
||||
attention_mask: Optional[torch.Tensor] = None,
|
||||
past_key_value: Optional[Tuple[torch.Tensor]] = None,
|
||||
output_attentions: Optional[bool] = False,
|
||||
use_cache: Optional[bool] = False,
|
||||
) -> Tuple[torch.FloatTensor, Optional[Tuple[torch.FloatTensor, torch.FloatTensor]]]:
|
||||
|
||||
residual = hidden_states
|
||||
|
||||
hidden_states = self.input_layernorm(hidden_states)
|
||||
|
||||
# Self Attention
|
||||
hidden_states, self_attn_weights, present_key_value = self.self_attn(
|
||||
hidden_states=hidden_states,
|
||||
attention_mask=attention_mask,
|
||||
past_key_value=past_key_value,
|
||||
output_attentions=output_attentions,
|
||||
use_cache=use_cache,
|
||||
)
|
||||
hidden_states = residual + hidden_states
|
||||
|
||||
# Fully Connected
|
||||
residual = hidden_states
|
||||
hidden_states = self.post_attention_layernorm(hidden_states)
|
||||
hidden_states = self.mlp(hidden_states)
|
||||
hidden_states = residual + hidden_states
|
||||
|
||||
outputs = (hidden_states,)
|
||||
|
||||
if use_cache:
|
||||
outputs += (present_key_value,)
|
||||
|
||||
return outputs
|
||||
|
||||
|
||||
class BaichuanPreTrainedModel(PreTrainedModel):
|
||||
config_class = BaichuanConfig
|
||||
base_model_prefix = "model"
|
||||
supports_gradient_checkpointing = True
|
||||
_no_split_modules = ["BaichuanLayer"]
|
||||
_keys_to_ignore_on_load_unexpected = [r"decoder\.version"]
|
||||
|
||||
def _init_weights(self, module):
|
||||
std = self.config.initializer_range
|
||||
if isinstance(module, torch.nn.Linear):
|
||||
module.weight.data.normal_(mean=0.0, std=std)
|
||||
if module.bias is not None:
|
||||
module.bias.data.zero_()
|
||||
elif isinstance(module, torch.nn.Embedding):
|
||||
module.weight.data.normal_(mean=0.0, std=std)
|
||||
if module.padding_idx is not None:
|
||||
module.weight.data[module.padding_idx].zero_()
|
||||
|
||||
def _set_gradient_checkpointing(self, module, value=False):
|
||||
if isinstance(module, BaichuanModel):
|
||||
module.gradient_checkpointing = value
|
||||
|
||||
|
||||
|
||||
class BaichuanModel(BaichuanPreTrainedModel):
|
||||
def __init__(self, config: BaichuanConfig):
|
||||
super().__init__(config)
|
||||
self.padding_idx = config.pad_token_id
|
||||
self.vocab_size = config.vocab_size
|
||||
self.n_head = config.num_attention_heads
|
||||
self.embed_tokens = torch.nn.Embedding(config.vocab_size, config.hidden_size, self.padding_idx)
|
||||
self.layers = torch.nn.ModuleList([BaichuanLayer(config) for _ in range(config.num_hidden_layers)])
|
||||
self.norm = RMSNorm(config.hidden_size, epsilon=config.rms_norm_eps)
|
||||
|
||||
self.gradient_checkpointing = config.gradient_checkpointing
|
||||
self.post_init()
|
||||
self.max_cache_pos = config.model_max_length
|
||||
self.first_run = True
|
||||
|
||||
def get_input_embeddings(self):
|
||||
return self.embed_tokens
|
||||
|
||||
def set_input_embeddings(self, value):
|
||||
self.embed_tokens = value
|
||||
|
||||
def get_alibi_mask(self, tensor, seq_length_with_past):
|
||||
if self.first_run:
|
||||
self.first_run = False
|
||||
self.register_buffer("future_mask", _gen_alibi_mask(self.n_head, self.max_cache_pos).to(tensor), persistent=False)
|
||||
if seq_length_with_past > self.max_cache_pos:
|
||||
self.max_cache_pos = seq_length_with_past
|
||||
self.register_buffer("future_mask", _gen_alibi_mask(self.n_head, self.max_cache_pos).to(tensor), persistent=False)
|
||||
mask = self.future_mask[:self.n_head, :seq_length_with_past, :seq_length_with_past]
|
||||
return mask
|
||||
|
||||
def forward(
|
||||
self,
|
||||
input_ids: torch.LongTensor = None,
|
||||
past_key_values: Optional[List[torch.FloatTensor]] = None,
|
||||
inputs_embeds: Optional[torch.FloatTensor] = None,
|
||||
use_cache: Optional[bool] = False,
|
||||
output_attentions: Optional[bool] = False,
|
||||
output_hidden_states: Optional[bool] = False,
|
||||
return_dict: Optional[bool] = True,
|
||||
) -> Union[Tuple, BaseModelOutputWithPast]:
|
||||
|
||||
|
||||
if input_ids is not None and inputs_embeds is not None:
|
||||
raise ValueError("You cannot provide both input_ids and inputs_embeds simultaneously")
|
||||
elif input_ids is not None:
|
||||
batch_size, seq_length = input_ids.shape
|
||||
elif inputs_embeds is not None:
|
||||
batch_size, seq_length, _ = inputs_embeds.shape
|
||||
else:
|
||||
raise ValueError("You need to provide input_ids or inputs_embeds")
|
||||
|
||||
seq_length_with_past = seq_length
|
||||
|
||||
if past_key_values is not None:
|
||||
past_key_values_length = past_key_values[0][0].shape[2]
|
||||
seq_length_with_past = seq_length_with_past + past_key_values_length
|
||||
|
||||
if inputs_embeds is None:
|
||||
inputs_embeds = self.embed_tokens(input_ids)
|
||||
|
||||
# embed positions
|
||||
attention_mask = self.get_alibi_mask(inputs_embeds, seq_length_with_past)
|
||||
|
||||
hidden_states = inputs_embeds
|
||||
|
||||
if self.gradient_checkpointing and self.training:
|
||||
if use_cache:
|
||||
logger.warning_once(
|
||||
"`use_cache=True` is incompatible with gradient checkpointing. Setting `use_cache=False`..."
|
||||
)
|
||||
use_cache = False
|
||||
|
||||
# decoder layers
|
||||
all_hidden_states = () if output_hidden_states else None
|
||||
all_self_attns = () if output_attentions else None
|
||||
next_decoder_cache = () if use_cache else None
|
||||
|
||||
for idx, decoder_layer in enumerate(self.layers):
|
||||
if output_hidden_states:
|
||||
all_hidden_states += (hidden_states,)
|
||||
|
||||
past_key_value = past_key_values[idx] if past_key_values is not None else None
|
||||
|
||||
if self.gradient_checkpointing and self.training:
|
||||
|
||||
def create_custom_forward(module):
|
||||
def custom_forward(*inputs):
|
||||
# None for past_key_value
|
||||
return module(*inputs, output_attentions, None)
|
||||
|
||||
return custom_forward
|
||||
|
||||
layer_outputs = torch.utils.checkpoint.checkpoint(
|
||||
create_custom_forward(decoder_layer),
|
||||
hidden_states,
|
||||
attention_mask,
|
||||
None,
|
||||
)
|
||||
else:
|
||||
layer_outputs = decoder_layer(
|
||||
hidden_states,
|
||||
attention_mask=attention_mask,
|
||||
past_key_value=past_key_value,
|
||||
output_attentions=output_attentions,
|
||||
use_cache=use_cache,
|
||||
)
|
||||
|
||||
hidden_states = layer_outputs[0]
|
||||
|
||||
if use_cache:
|
||||
next_decoder_cache += (layer_outputs[2 if output_attentions else 1],)
|
||||
|
||||
if output_attentions:
|
||||
all_self_attns += (layer_outputs[1],)
|
||||
|
||||
hidden_states = self.norm(hidden_states)
|
||||
|
||||
# add hidden states from the last decoder layer
|
||||
if output_hidden_states:
|
||||
all_hidden_states += (hidden_states,)
|
||||
|
||||
next_cache = next_decoder_cache if use_cache else None
|
||||
if not return_dict:
|
||||
return tuple(v for v in [hidden_states, next_cache, all_hidden_states, all_self_attns] if v is not None)
|
||||
return BaseModelOutputWithPast(
|
||||
last_hidden_state=hidden_states,
|
||||
past_key_values=next_cache,
|
||||
hidden_states=all_hidden_states,
|
||||
attentions=all_self_attns,
|
||||
)
|
||||
|
||||
|
||||
class BaichuanForCausalLM(BaichuanPreTrainedModel):
|
||||
def __init__(self, config):
|
||||
super().__init__(config)
|
||||
self.model = BaichuanModel(config)
|
||||
self.lm_head = torch.nn.Linear(config.hidden_size, config.vocab_size, bias=False)
|
||||
|
||||
# Initialize weights and apply final processing
|
||||
self.post_init()
|
||||
|
||||
def forward(
|
||||
self,
|
||||
input_ids: torch.LongTensor = None,
|
||||
past_key_values: Optional[List[torch.FloatTensor]] = None,
|
||||
inputs_embeds: Optional[torch.FloatTensor] = None,
|
||||
labels: Optional[torch.LongTensor] = None,
|
||||
use_cache: Optional[bool] = None,
|
||||
output_attentions: Optional[bool] = False,
|
||||
output_hidden_states: Optional[bool] = False,
|
||||
return_dict: Optional[bool] = True,
|
||||
**kwargs
|
||||
) -> Union[Tuple, CausalLMOutputWithPast]:
|
||||
|
||||
|
||||
# decoder outputs consists of (dec_features, layer_state, dec_hidden, dec_attn)
|
||||
outputs = self.model(
|
||||
input_ids=input_ids,
|
||||
past_key_values=past_key_values,
|
||||
inputs_embeds=inputs_embeds,
|
||||
use_cache=use_cache,
|
||||
output_attentions=output_attentions,
|
||||
output_hidden_states=output_hidden_states,
|
||||
return_dict=return_dict,
|
||||
)
|
||||
|
||||
hidden_states = outputs[0]
|
||||
logits = self.lm_head(hidden_states)
|
||||
|
||||
loss = None
|
||||
if labels is not None:
|
||||
# Shift so that tokens < n predict n
|
||||
shift_logits = logits[..., :-1, :].contiguous()
|
||||
shift_labels = labels[..., 1:].contiguous()
|
||||
# Flatten the tokens
|
||||
loss_fct = CrossEntropyLoss()
|
||||
shift_logits = shift_logits.view(-1, self.config.vocab_size)
|
||||
shift_labels = shift_labels.view(-1)
|
||||
# Enable model parallelism
|
||||
shift_labels = shift_labels.to(shift_logits.device)
|
||||
loss = loss_fct(shift_logits, shift_labels)
|
||||
|
||||
if not return_dict:
|
||||
output = (logits,) + outputs[1:]
|
||||
return (loss,) + output if loss is not None else output
|
||||
|
||||
return CausalLMOutputWithPast(
|
||||
loss=loss,
|
||||
logits=logits,
|
||||
past_key_values=outputs.past_key_values,
|
||||
hidden_states=outputs.hidden_states,
|
||||
attentions=outputs.attentions,
|
||||
)
|
||||
|
||||
def prepare_inputs_for_generation(
|
||||
self, input_ids, past_key_values=None, attention_mask=None, inputs_embeds=None, **kwargs
|
||||
):
|
||||
if past_key_values:
|
||||
input_ids = input_ids[:, -1:]
|
||||
|
||||
# if `inputs_embeds` are passed, we only want to use them in the 1st generation step
|
||||
if inputs_embeds is not None and past_key_values is None:
|
||||
model_inputs = {"inputs_embeds": inputs_embeds}
|
||||
else:
|
||||
model_inputs = {"input_ids": input_ids}
|
||||
|
||||
model_inputs.update(
|
||||
{
|
||||
"past_key_values": past_key_values,
|
||||
"use_cache": kwargs.get("use_cache"),
|
||||
}
|
||||
)
|
||||
return model_inputs
|
||||
|
||||
@staticmethod
|
||||
def _reorder_cache(past_key_values, beam_idx):
|
||||
return tuple(
|
||||
tuple(past_state.index_select(0, beam_idx) for past_state in layer_past)
|
||||
for layer_past in past_key_values
|
||||
)
|
||||
|
||||
|
||||
def quantize(self, bits: int):
|
||||
try:
|
||||
from .quantizer import QLinear
|
||||
except ImportError:
|
||||
raise ImportError(
|
||||
f"Needs QLinear to run quantize."
|
||||
)
|
||||
|
||||
for layer in self.model.layers:
|
||||
layer.self_attn.W_pack = QLinear(
|
||||
bits=bits,
|
||||
weight=layer.self_attn.W_pack.weight,
|
||||
bias = None,
|
||||
)
|
||||
layer.self_attn.o_proj = QLinear(
|
||||
bits=bits,
|
||||
weight=layer.self_attn.o_proj.weight,
|
||||
bias = None,
|
||||
)
|
||||
layer.mlp.gate_proj = QLinear(
|
||||
bits=bits,
|
||||
weight=layer.mlp.gate_proj.weight,
|
||||
bias = None,
|
||||
)
|
||||
layer.mlp.down_proj = QLinear(
|
||||
bits=bits,
|
||||
weight=layer.mlp.down_proj.weight,
|
||||
bias = None,
|
||||
)
|
||||
layer.mlp.up_proj = QLinear(
|
||||
bits=bits,
|
||||
weight=layer.mlp.up_proj.weight,
|
||||
bias = None,
|
||||
)
|
||||
return self
|
||||
|
||||
def _build_chat_input(self, tokenizer, messages: List[dict], max_new_tokens: int=0):
|
||||
max_new_tokens = max_new_tokens or self.generation_config.max_new_tokens
|
||||
max_input_tokens = self.config.model_max_length - max_new_tokens
|
||||
max_input_tokens = max(self.config.model_max_length // 2, max_input_tokens)
|
||||
total_input, round_input = [], []
|
||||
for i, message in enumerate(messages[::-1]):
|
||||
content_tokens = tokenizer.encode(message['content'])
|
||||
if message['role'] == 'user':
|
||||
round_input = [self.generation_config.user_token_id] + content_tokens + round_input
|
||||
if total_input and len(total_input) + len(round_input) > max_input_tokens:
|
||||
break
|
||||
else:
|
||||
total_input = round_input + total_input
|
||||
if len(total_input) >= max_input_tokens:
|
||||
break
|
||||
else:
|
||||
round_input = []
|
||||
elif message['role'] == 'assistant':
|
||||
round_input = [
|
||||
self.generation_config.assistant_token_id
|
||||
] + content_tokens + [
|
||||
self.generation_config.eos_token_id
|
||||
] + round_input
|
||||
else:
|
||||
raise ValueError(f"message role not supported yet: {message['role']}")
|
||||
total_input = total_input[-max_input_tokens:] # truncate left
|
||||
total_input.append(self.generation_config.assistant_token_id)
|
||||
total_input = torch.LongTensor([total_input]).to(self.device)
|
||||
return total_input
|
||||
|
||||
@torch.no_grad()
|
||||
def chat(self, tokenizer, messages: List[dict], stream=False,
|
||||
generation_config: Optional[GenerationConfig]=None):
|
||||
generation_config = generation_config or self.generation_config
|
||||
input_ids = self._build_chat_input(tokenizer, messages, generation_config.max_new_tokens)
|
||||
if stream:
|
||||
from transformers_stream_generator.main import NewGenerationMixin, StreamGenerationConfig
|
||||
self.__class__.generate = NewGenerationMixin.generate
|
||||
self.__class__.sample_stream = NewGenerationMixin.sample_stream
|
||||
stream_config = StreamGenerationConfig(**generation_config.to_dict(), do_stream=True)
|
||||
|
||||
def stream_generator():
|
||||
outputs = []
|
||||
for token in self.generate(input_ids, generation_config=stream_config):
|
||||
outputs.append(token.item())
|
||||
yield tokenizer.decode(outputs, skip_special_tokens=True)
|
||||
|
||||
return stream_generator()
|
||||
else:
|
||||
self.__class__.generate = PreTrainedModel.generate # disable stream
|
||||
outputs = self.generate(input_ids, generation_config=generation_config)
|
||||
response = tokenizer.decode(outputs[0][len(input_ids[0]):], skip_special_tokens=True)
|
||||
return response
|
Binary file not shown.
Binary file not shown.
Binary file not shown.
|
@ -0,0 +1,290 @@
|
|||
{
|
||||
"metadata": {
|
||||
"total_size": 26529802240
|
||||
},
|
||||
"weight_map": {
|
||||
"lm_head.weight": "pytorch_model-00003-of-00003.bin",
|
||||
"model.embed_tokens.weight": "pytorch_model-00001-of-00003.bin",
|
||||
"model.layers.0.input_layernorm.weight": "pytorch_model-00001-of-00003.bin",
|
||||
"model.layers.0.mlp.down_proj.weight": "pytorch_model-00001-of-00003.bin",
|
||||
"model.layers.0.mlp.gate_proj.weight": "pytorch_model-00001-of-00003.bin",
|
||||
"model.layers.0.mlp.up_proj.weight": "pytorch_model-00001-of-00003.bin",
|
||||
"model.layers.0.post_attention_layernorm.weight": "pytorch_model-00001-of-00003.bin",
|
||||
"model.layers.0.self_attn.W_pack.weight": "pytorch_model-00001-of-00003.bin",
|
||||
"model.layers.0.self_attn.o_proj.weight": "pytorch_model-00001-of-00003.bin",
|
||||
"model.layers.1.input_layernorm.weight": "pytorch_model-00001-of-00003.bin",
|
||||
"model.layers.1.mlp.down_proj.weight": "pytorch_model-00001-of-00003.bin",
|
||||
"model.layers.1.mlp.gate_proj.weight": "pytorch_model-00001-of-00003.bin",
|
||||
"model.layers.1.mlp.up_proj.weight": "pytorch_model-00001-of-00003.bin",
|
||||
"model.layers.1.post_attention_layernorm.weight": "pytorch_model-00001-of-00003.bin",
|
||||
"model.layers.1.self_attn.W_pack.weight": "pytorch_model-00001-of-00003.bin",
|
||||
"model.layers.1.self_attn.o_proj.weight": "pytorch_model-00001-of-00003.bin",
|
||||
"model.layers.10.input_layernorm.weight": "pytorch_model-00001-of-00003.bin",
|
||||
"model.layers.10.mlp.down_proj.weight": "pytorch_model-00001-of-00003.bin",
|
||||
"model.layers.10.mlp.gate_proj.weight": "pytorch_model-00001-of-00003.bin",
|
||||
"model.layers.10.mlp.up_proj.weight": "pytorch_model-00001-of-00003.bin",
|
||||
"model.layers.10.post_attention_layernorm.weight": "pytorch_model-00001-of-00003.bin",
|
||||
"model.layers.10.self_attn.W_pack.weight": "pytorch_model-00001-of-00003.bin",
|
||||
"model.layers.10.self_attn.o_proj.weight": "pytorch_model-00001-of-00003.bin",
|
||||
"model.layers.11.input_layernorm.weight": "pytorch_model-00001-of-00003.bin",
|
||||
"model.layers.11.mlp.down_proj.weight": "pytorch_model-00001-of-00003.bin",
|
||||
"model.layers.11.mlp.gate_proj.weight": "pytorch_model-00001-of-00003.bin",
|
||||
"model.layers.11.mlp.up_proj.weight": "pytorch_model-00001-of-00003.bin",
|
||||
"model.layers.11.post_attention_layernorm.weight": "pytorch_model-00001-of-00003.bin",
|
||||
"model.layers.11.self_attn.W_pack.weight": "pytorch_model-00001-of-00003.bin",
|
||||
"model.layers.11.self_attn.o_proj.weight": "pytorch_model-00001-of-00003.bin",
|
||||
"model.layers.12.input_layernorm.weight": "pytorch_model-00001-of-00003.bin",
|
||||
"model.layers.12.mlp.down_proj.weight": "pytorch_model-00001-of-00003.bin",
|
||||
"model.layers.12.mlp.gate_proj.weight": "pytorch_model-00001-of-00003.bin",
|
||||
"model.layers.12.mlp.up_proj.weight": "pytorch_model-00001-of-00003.bin",
|
||||
"model.layers.12.post_attention_layernorm.weight": "pytorch_model-00001-of-00003.bin",
|
||||
"model.layers.12.self_attn.W_pack.weight": "pytorch_model-00001-of-00003.bin",
|
||||
"model.layers.12.self_attn.o_proj.weight": "pytorch_model-00001-of-00003.bin",
|
||||
"model.layers.13.input_layernorm.weight": "pytorch_model-00001-of-00003.bin",
|
||||
"model.layers.13.mlp.down_proj.weight": "pytorch_model-00001-of-00003.bin",
|
||||
"model.layers.13.mlp.gate_proj.weight": "pytorch_model-00001-of-00003.bin",
|
||||
"model.layers.13.mlp.up_proj.weight": "pytorch_model-00001-of-00003.bin",
|
||||
"model.layers.13.post_attention_layernorm.weight": "pytorch_model-00001-of-00003.bin",
|
||||
"model.layers.13.self_attn.W_pack.weight": "pytorch_model-00001-of-00003.bin",
|
||||
"model.layers.13.self_attn.o_proj.weight": "pytorch_model-00001-of-00003.bin",
|
||||
"model.layers.14.input_layernorm.weight": "pytorch_model-00002-of-00003.bin",
|
||||
"model.layers.14.mlp.down_proj.weight": "pytorch_model-00001-of-00003.bin",
|
||||
"model.layers.14.mlp.gate_proj.weight": "pytorch_model-00001-of-00003.bin",
|
||||
"model.layers.14.mlp.up_proj.weight": "pytorch_model-00002-of-00003.bin",
|
||||
"model.layers.14.post_attention_layernorm.weight": "pytorch_model-00002-of-00003.bin",
|
||||
"model.layers.14.self_attn.W_pack.weight": "pytorch_model-00001-of-00003.bin",
|
||||
"model.layers.14.self_attn.o_proj.weight": "pytorch_model-00001-of-00003.bin",
|
||||
"model.layers.15.input_layernorm.weight": "pytorch_model-00002-of-00003.bin",
|
||||
"model.layers.15.mlp.down_proj.weight": "pytorch_model-00002-of-00003.bin",
|
||||
"model.layers.15.mlp.gate_proj.weight": "pytorch_model-00002-of-00003.bin",
|
||||
"model.layers.15.mlp.up_proj.weight": "pytorch_model-00002-of-00003.bin",
|
||||
"model.layers.15.post_attention_layernorm.weight": "pytorch_model-00002-of-00003.bin",
|
||||
"model.layers.15.self_attn.W_pack.weight": "pytorch_model-00002-of-00003.bin",
|
||||
"model.layers.15.self_attn.o_proj.weight": "pytorch_model-00002-of-00003.bin",
|
||||
"model.layers.16.input_layernorm.weight": "pytorch_model-00002-of-00003.bin",
|
||||
"model.layers.16.mlp.down_proj.weight": "pytorch_model-00002-of-00003.bin",
|
||||
"model.layers.16.mlp.gate_proj.weight": "pytorch_model-00002-of-00003.bin",
|
||||
"model.layers.16.mlp.up_proj.weight": "pytorch_model-00002-of-00003.bin",
|
||||
"model.layers.16.post_attention_layernorm.weight": "pytorch_model-00002-of-00003.bin",
|
||||
"model.layers.16.self_attn.W_pack.weight": "pytorch_model-00002-of-00003.bin",
|
||||
"model.layers.16.self_attn.o_proj.weight": "pytorch_model-00002-of-00003.bin",
|
||||
"model.layers.17.input_layernorm.weight": "pytorch_model-00002-of-00003.bin",
|
||||
"model.layers.17.mlp.down_proj.weight": "pytorch_model-00002-of-00003.bin",
|
||||
"model.layers.17.mlp.gate_proj.weight": "pytorch_model-00002-of-00003.bin",
|
||||
"model.layers.17.mlp.up_proj.weight": "pytorch_model-00002-of-00003.bin",
|
||||
"model.layers.17.post_attention_layernorm.weight": "pytorch_model-00002-of-00003.bin",
|
||||
"model.layers.17.self_attn.W_pack.weight": "pytorch_model-00002-of-00003.bin",
|
||||
"model.layers.17.self_attn.o_proj.weight": "pytorch_model-00002-of-00003.bin",
|
||||
"model.layers.18.input_layernorm.weight": "pytorch_model-00002-of-00003.bin",
|
||||
"model.layers.18.mlp.down_proj.weight": "pytorch_model-00002-of-00003.bin",
|
||||
"model.layers.18.mlp.gate_proj.weight": "pytorch_model-00002-of-00003.bin",
|
||||
"model.layers.18.mlp.up_proj.weight": "pytorch_model-00002-of-00003.bin",
|
||||
"model.layers.18.post_attention_layernorm.weight": "pytorch_model-00002-of-00003.bin",
|
||||
"model.layers.18.self_attn.W_pack.weight": "pytorch_model-00002-of-00003.bin",
|
||||
"model.layers.18.self_attn.o_proj.weight": "pytorch_model-00002-of-00003.bin",
|
||||
"model.layers.19.input_layernorm.weight": "pytorch_model-00002-of-00003.bin",
|
||||
"model.layers.19.mlp.down_proj.weight": "pytorch_model-00002-of-00003.bin",
|
||||
"model.layers.19.mlp.gate_proj.weight": "pytorch_model-00002-of-00003.bin",
|
||||
"model.layers.19.mlp.up_proj.weight": "pytorch_model-00002-of-00003.bin",
|
||||
"model.layers.19.post_attention_layernorm.weight": "pytorch_model-00002-of-00003.bin",
|
||||
"model.layers.19.self_attn.W_pack.weight": "pytorch_model-00002-of-00003.bin",
|
||||
"model.layers.19.self_attn.o_proj.weight": "pytorch_model-00002-of-00003.bin",
|
||||
"model.layers.2.input_layernorm.weight": "pytorch_model-00001-of-00003.bin",
|
||||
"model.layers.2.mlp.down_proj.weight": "pytorch_model-00001-of-00003.bin",
|
||||
"model.layers.2.mlp.gate_proj.weight": "pytorch_model-00001-of-00003.bin",
|
||||
"model.layers.2.mlp.up_proj.weight": "pytorch_model-00001-of-00003.bin",
|
||||
"model.layers.2.post_attention_layernorm.weight": "pytorch_model-00001-of-00003.bin",
|
||||
"model.layers.2.self_attn.W_pack.weight": "pytorch_model-00001-of-00003.bin",
|
||||
"model.layers.2.self_attn.o_proj.weight": "pytorch_model-00001-of-00003.bin",
|
||||
"model.layers.20.input_layernorm.weight": "pytorch_model-00002-of-00003.bin",
|
||||
"model.layers.20.mlp.down_proj.weight": "pytorch_model-00002-of-00003.bin",
|
||||
"model.layers.20.mlp.gate_proj.weight": "pytorch_model-00002-of-00003.bin",
|
||||
"model.layers.20.mlp.up_proj.weight": "pytorch_model-00002-of-00003.bin",
|
||||
"model.layers.20.post_attention_layernorm.weight": "pytorch_model-00002-of-00003.bin",
|
||||
"model.layers.20.self_attn.W_pack.weight": "pytorch_model-00002-of-00003.bin",
|
||||
"model.layers.20.self_attn.o_proj.weight": "pytorch_model-00002-of-00003.bin",
|
||||
"model.layers.21.input_layernorm.weight": "pytorch_model-00002-of-00003.bin",
|
||||
"model.layers.21.mlp.down_proj.weight": "pytorch_model-00002-of-00003.bin",
|
||||
"model.layers.21.mlp.gate_proj.weight": "pytorch_model-00002-of-00003.bin",
|
||||
"model.layers.21.mlp.up_proj.weight": "pytorch_model-00002-of-00003.bin",
|
||||
"model.layers.21.post_attention_layernorm.weight": "pytorch_model-00002-of-00003.bin",
|
||||
"model.layers.21.self_attn.W_pack.weight": "pytorch_model-00002-of-00003.bin",
|
||||
"model.layers.21.self_attn.o_proj.weight": "pytorch_model-00002-of-00003.bin",
|
||||
"model.layers.22.input_layernorm.weight": "pytorch_model-00002-of-00003.bin",
|
||||
"model.layers.22.mlp.down_proj.weight": "pytorch_model-00002-of-00003.bin",
|
||||
"model.layers.22.mlp.gate_proj.weight": "pytorch_model-00002-of-00003.bin",
|
||||
"model.layers.22.mlp.up_proj.weight": "pytorch_model-00002-of-00003.bin",
|
||||
"model.layers.22.post_attention_layernorm.weight": "pytorch_model-00002-of-00003.bin",
|
||||
"model.layers.22.self_attn.W_pack.weight": "pytorch_model-00002-of-00003.bin",
|
||||
"model.layers.22.self_attn.o_proj.weight": "pytorch_model-00002-of-00003.bin",
|
||||
"model.layers.23.input_layernorm.weight": "pytorch_model-00002-of-00003.bin",
|
||||
"model.layers.23.mlp.down_proj.weight": "pytorch_model-00002-of-00003.bin",
|
||||
"model.layers.23.mlp.gate_proj.weight": "pytorch_model-00002-of-00003.bin",
|
||||
"model.layers.23.mlp.up_proj.weight": "pytorch_model-00002-of-00003.bin",
|
||||
"model.layers.23.post_attention_layernorm.weight": "pytorch_model-00002-of-00003.bin",
|
||||
"model.layers.23.self_attn.W_pack.weight": "pytorch_model-00002-of-00003.bin",
|
||||
"model.layers.23.self_attn.o_proj.weight": "pytorch_model-00002-of-00003.bin",
|
||||
"model.layers.24.input_layernorm.weight": "pytorch_model-00002-of-00003.bin",
|
||||
"model.layers.24.mlp.down_proj.weight": "pytorch_model-00002-of-00003.bin",
|
||||
"model.layers.24.mlp.gate_proj.weight": "pytorch_model-00002-of-00003.bin",
|
||||
"model.layers.24.mlp.up_proj.weight": "pytorch_model-00002-of-00003.bin",
|
||||
"model.layers.24.post_attention_layernorm.weight": "pytorch_model-00002-of-00003.bin",
|
||||
"model.layers.24.self_attn.W_pack.weight": "pytorch_model-00002-of-00003.bin",
|
||||
"model.layers.24.self_attn.o_proj.weight": "pytorch_model-00002-of-00003.bin",
|
||||
"model.layers.25.input_layernorm.weight": "pytorch_model-00002-of-00003.bin",
|
||||
"model.layers.25.mlp.down_proj.weight": "pytorch_model-00002-of-00003.bin",
|
||||
"model.layers.25.mlp.gate_proj.weight": "pytorch_model-00002-of-00003.bin",
|
||||
"model.layers.25.mlp.up_proj.weight": "pytorch_model-00002-of-00003.bin",
|
||||
"model.layers.25.post_attention_layernorm.weight": "pytorch_model-00002-of-00003.bin",
|
||||
"model.layers.25.self_attn.W_pack.weight": "pytorch_model-00002-of-00003.bin",
|
||||
"model.layers.25.self_attn.o_proj.weight": "pytorch_model-00002-of-00003.bin",
|
||||
"model.layers.26.input_layernorm.weight": "pytorch_model-00002-of-00003.bin",
|
||||
"model.layers.26.mlp.down_proj.weight": "pytorch_model-00002-of-00003.bin",
|
||||
"model.layers.26.mlp.gate_proj.weight": "pytorch_model-00002-of-00003.bin",
|
||||
"model.layers.26.mlp.up_proj.weight": "pytorch_model-00002-of-00003.bin",
|
||||
"model.layers.26.post_attention_layernorm.weight": "pytorch_model-00002-of-00003.bin",
|
||||
"model.layers.26.self_attn.W_pack.weight": "pytorch_model-00002-of-00003.bin",
|
||||
"model.layers.26.self_attn.o_proj.weight": "pytorch_model-00002-of-00003.bin",
|
||||
"model.layers.27.input_layernorm.weight": "pytorch_model-00002-of-00003.bin",
|
||||
"model.layers.27.mlp.down_proj.weight": "pytorch_model-00002-of-00003.bin",
|
||||
"model.layers.27.mlp.gate_proj.weight": "pytorch_model-00002-of-00003.bin",
|
||||
"model.layers.27.mlp.up_proj.weight": "pytorch_model-00002-of-00003.bin",
|
||||
"model.layers.27.post_attention_layernorm.weight": "pytorch_model-00002-of-00003.bin",
|
||||
"model.layers.27.self_attn.W_pack.weight": "pytorch_model-00002-of-00003.bin",
|
||||
"model.layers.27.self_attn.o_proj.weight": "pytorch_model-00002-of-00003.bin",
|
||||
"model.layers.28.input_layernorm.weight": "pytorch_model-00002-of-00003.bin",
|
||||
"model.layers.28.mlp.down_proj.weight": "pytorch_model-00002-of-00003.bin",
|
||||
"model.layers.28.mlp.gate_proj.weight": "pytorch_model-00002-of-00003.bin",
|
||||
"model.layers.28.mlp.up_proj.weight": "pytorch_model-00002-of-00003.bin",
|
||||
"model.layers.28.post_attention_layernorm.weight": "pytorch_model-00002-of-00003.bin",
|
||||
"model.layers.28.self_attn.W_pack.weight": "pytorch_model-00002-of-00003.bin",
|
||||
"model.layers.28.self_attn.o_proj.weight": "pytorch_model-00002-of-00003.bin",
|
||||
"model.layers.29.input_layernorm.weight": "pytorch_model-00002-of-00003.bin",
|
||||
"model.layers.29.mlp.down_proj.weight": "pytorch_model-00002-of-00003.bin",
|
||||
"model.layers.29.mlp.gate_proj.weight": "pytorch_model-00002-of-00003.bin",
|
||||
"model.layers.29.mlp.up_proj.weight": "pytorch_model-00002-of-00003.bin",
|
||||
"model.layers.29.post_attention_layernorm.weight": "pytorch_model-00002-of-00003.bin",
|
||||
"model.layers.29.self_attn.W_pack.weight": "pytorch_model-00002-of-00003.bin",
|
||||
"model.layers.29.self_attn.o_proj.weight": "pytorch_model-00002-of-00003.bin",
|
||||
"model.layers.3.input_layernorm.weight": "pytorch_model-00001-of-00003.bin",
|
||||
"model.layers.3.mlp.down_proj.weight": "pytorch_model-00001-of-00003.bin",
|
||||
"model.layers.3.mlp.gate_proj.weight": "pytorch_model-00001-of-00003.bin",
|
||||
"model.layers.3.mlp.up_proj.weight": "pytorch_model-00001-of-00003.bin",
|
||||
"model.layers.3.post_attention_layernorm.weight": "pytorch_model-00001-of-00003.bin",
|
||||
"model.layers.3.self_attn.W_pack.weight": "pytorch_model-00001-of-00003.bin",
|
||||
"model.layers.3.self_attn.o_proj.weight": "pytorch_model-00001-of-00003.bin",
|
||||
"model.layers.30.input_layernorm.weight": "pytorch_model-00003-of-00003.bin",
|
||||
"model.layers.30.mlp.down_proj.weight": "pytorch_model-00003-of-00003.bin",
|
||||
"model.layers.30.mlp.gate_proj.weight": "pytorch_model-00002-of-00003.bin",
|
||||
"model.layers.30.mlp.up_proj.weight": "pytorch_model-00003-of-00003.bin",
|
||||
"model.layers.30.post_attention_layernorm.weight": "pytorch_model-00003-of-00003.bin",
|
||||
"model.layers.30.self_attn.W_pack.weight": "pytorch_model-00002-of-00003.bin",
|
||||
"model.layers.30.self_attn.o_proj.weight": "pytorch_model-00002-of-00003.bin",
|
||||
"model.layers.31.input_layernorm.weight": "pytorch_model-00003-of-00003.bin",
|
||||
"model.layers.31.mlp.down_proj.weight": "pytorch_model-00003-of-00003.bin",
|
||||
"model.layers.31.mlp.gate_proj.weight": "pytorch_model-00003-of-00003.bin",
|
||||
"model.layers.31.mlp.up_proj.weight": "pytorch_model-00003-of-00003.bin",
|
||||
"model.layers.31.post_attention_layernorm.weight": "pytorch_model-00003-of-00003.bin",
|
||||
"model.layers.31.self_attn.W_pack.weight": "pytorch_model-00003-of-00003.bin",
|
||||
"model.layers.31.self_attn.o_proj.weight": "pytorch_model-00003-of-00003.bin",
|
||||
"model.layers.32.input_layernorm.weight": "pytorch_model-00003-of-00003.bin",
|
||||
"model.layers.32.mlp.down_proj.weight": "pytorch_model-00003-of-00003.bin",
|
||||
"model.layers.32.mlp.gate_proj.weight": "pytorch_model-00003-of-00003.bin",
|
||||
"model.layers.32.mlp.up_proj.weight": "pytorch_model-00003-of-00003.bin",
|
||||
"model.layers.32.post_attention_layernorm.weight": "pytorch_model-00003-of-00003.bin",
|
||||
"model.layers.32.self_attn.W_pack.weight": "pytorch_model-00003-of-00003.bin",
|
||||
"model.layers.32.self_attn.o_proj.weight": "pytorch_model-00003-of-00003.bin",
|
||||
"model.layers.33.input_layernorm.weight": "pytorch_model-00003-of-00003.bin",
|
||||
"model.layers.33.mlp.down_proj.weight": "pytorch_model-00003-of-00003.bin",
|
||||
"model.layers.33.mlp.gate_proj.weight": "pytorch_model-00003-of-00003.bin",
|
||||
"model.layers.33.mlp.up_proj.weight": "pytorch_model-00003-of-00003.bin",
|
||||
"model.layers.33.post_attention_layernorm.weight": "pytorch_model-00003-of-00003.bin",
|
||||
"model.layers.33.self_attn.W_pack.weight": "pytorch_model-00003-of-00003.bin",
|
||||
"model.layers.33.self_attn.o_proj.weight": "pytorch_model-00003-of-00003.bin",
|
||||
"model.layers.34.input_layernorm.weight": "pytorch_model-00003-of-00003.bin",
|
||||
"model.layers.34.mlp.down_proj.weight": "pytorch_model-00003-of-00003.bin",
|
||||
"model.layers.34.mlp.gate_proj.weight": "pytorch_model-00003-of-00003.bin",
|
||||
"model.layers.34.mlp.up_proj.weight": "pytorch_model-00003-of-00003.bin",
|
||||
"model.layers.34.post_attention_layernorm.weight": "pytorch_model-00003-of-00003.bin",
|
||||
"model.layers.34.self_attn.W_pack.weight": "pytorch_model-00003-of-00003.bin",
|
||||
"model.layers.34.self_attn.o_proj.weight": "pytorch_model-00003-of-00003.bin",
|
||||
"model.layers.35.input_layernorm.weight": "pytorch_model-00003-of-00003.bin",
|
||||
"model.layers.35.mlp.down_proj.weight": "pytorch_model-00003-of-00003.bin",
|
||||
"model.layers.35.mlp.gate_proj.weight": "pytorch_model-00003-of-00003.bin",
|
||||
"model.layers.35.mlp.up_proj.weight": "pytorch_model-00003-of-00003.bin",
|
||||
"model.layers.35.post_attention_layernorm.weight": "pytorch_model-00003-of-00003.bin",
|
||||
"model.layers.35.self_attn.W_pack.weight": "pytorch_model-00003-of-00003.bin",
|
||||
"model.layers.35.self_attn.o_proj.weight": "pytorch_model-00003-of-00003.bin",
|
||||
"model.layers.36.input_layernorm.weight": "pytorch_model-00003-of-00003.bin",
|
||||
"model.layers.36.mlp.down_proj.weight": "pytorch_model-00003-of-00003.bin",
|
||||
"model.layers.36.mlp.gate_proj.weight": "pytorch_model-00003-of-00003.bin",
|
||||
"model.layers.36.mlp.up_proj.weight": "pytorch_model-00003-of-00003.bin",
|
||||
"model.layers.36.post_attention_layernorm.weight": "pytorch_model-00003-of-00003.bin",
|
||||
"model.layers.36.self_attn.W_pack.weight": "pytorch_model-00003-of-00003.bin",
|
||||
"model.layers.36.self_attn.o_proj.weight": "pytorch_model-00003-of-00003.bin",
|
||||
"model.layers.37.input_layernorm.weight": "pytorch_model-00003-of-00003.bin",
|
||||
"model.layers.37.mlp.down_proj.weight": "pytorch_model-00003-of-00003.bin",
|
||||
"model.layers.37.mlp.gate_proj.weight": "pytorch_model-00003-of-00003.bin",
|
||||
"model.layers.37.mlp.up_proj.weight": "pytorch_model-00003-of-00003.bin",
|
||||
"model.layers.37.post_attention_layernorm.weight": "pytorch_model-00003-of-00003.bin",
|
||||
"model.layers.37.self_attn.W_pack.weight": "pytorch_model-00003-of-00003.bin",
|
||||
"model.layers.37.self_attn.o_proj.weight": "pytorch_model-00003-of-00003.bin",
|
||||
"model.layers.38.input_layernorm.weight": "pytorch_model-00003-of-00003.bin",
|
||||
"model.layers.38.mlp.down_proj.weight": "pytorch_model-00003-of-00003.bin",
|
||||
"model.layers.38.mlp.gate_proj.weight": "pytorch_model-00003-of-00003.bin",
|
||||
"model.layers.38.mlp.up_proj.weight": "pytorch_model-00003-of-00003.bin",
|
||||
"model.layers.38.post_attention_layernorm.weight": "pytorch_model-00003-of-00003.bin",
|
||||
"model.layers.38.self_attn.W_pack.weight": "pytorch_model-00003-of-00003.bin",
|
||||
"model.layers.38.self_attn.o_proj.weight": "pytorch_model-00003-of-00003.bin",
|
||||
"model.layers.39.input_layernorm.weight": "pytorch_model-00003-of-00003.bin",
|
||||
"model.layers.39.mlp.down_proj.weight": "pytorch_model-00003-of-00003.bin",
|
||||
"model.layers.39.mlp.gate_proj.weight": "pytorch_model-00003-of-00003.bin",
|
||||
"model.layers.39.mlp.up_proj.weight": "pytorch_model-00003-of-00003.bin",
|
||||
"model.layers.39.post_attention_layernorm.weight": "pytorch_model-00003-of-00003.bin",
|
||||
"model.layers.39.self_attn.W_pack.weight": "pytorch_model-00003-of-00003.bin",
|
||||
"model.layers.39.self_attn.o_proj.weight": "pytorch_model-00003-of-00003.bin",
|
||||
"model.layers.4.input_layernorm.weight": "pytorch_model-00001-of-00003.bin",
|
||||
"model.layers.4.mlp.down_proj.weight": "pytorch_model-00001-of-00003.bin",
|
||||
"model.layers.4.mlp.gate_proj.weight": "pytorch_model-00001-of-00003.bin",
|
||||
"model.layers.4.mlp.up_proj.weight": "pytorch_model-00001-of-00003.bin",
|
||||
"model.layers.4.post_attention_layernorm.weight": "pytorch_model-00001-of-00003.bin",
|
||||
"model.layers.4.self_attn.W_pack.weight": "pytorch_model-00001-of-00003.bin",
|
||||
"model.layers.4.self_attn.o_proj.weight": "pytorch_model-00001-of-00003.bin",
|
||||
"model.layers.5.input_layernorm.weight": "pytorch_model-00001-of-00003.bin",
|
||||
"model.layers.5.mlp.down_proj.weight": "pytorch_model-00001-of-00003.bin",
|
||||
"model.layers.5.mlp.gate_proj.weight": "pytorch_model-00001-of-00003.bin",
|
||||
"model.layers.5.mlp.up_proj.weight": "pytorch_model-00001-of-00003.bin",
|
||||
"model.layers.5.post_attention_layernorm.weight": "pytorch_model-00001-of-00003.bin",
|
||||
"model.layers.5.self_attn.W_pack.weight": "pytorch_model-00001-of-00003.bin",
|
||||
"model.layers.5.self_attn.o_proj.weight": "pytorch_model-00001-of-00003.bin",
|
||||
"model.layers.6.input_layernorm.weight": "pytorch_model-00001-of-00003.bin",
|
||||
"model.layers.6.mlp.down_proj.weight": "pytorch_model-00001-of-00003.bin",
|
||||
"model.layers.6.mlp.gate_proj.weight": "pytorch_model-00001-of-00003.bin",
|
||||
"model.layers.6.mlp.up_proj.weight": "pytorch_model-00001-of-00003.bin",
|
||||
"model.layers.6.post_attention_layernorm.weight": "pytorch_model-00001-of-00003.bin",
|
||||
"model.layers.6.self_attn.W_pack.weight": "pytorch_model-00001-of-00003.bin",
|
||||
"model.layers.6.self_attn.o_proj.weight": "pytorch_model-00001-of-00003.bin",
|
||||
"model.layers.7.input_layernorm.weight": "pytorch_model-00001-of-00003.bin",
|
||||
"model.layers.7.mlp.down_proj.weight": "pytorch_model-00001-of-00003.bin",
|
||||
"model.layers.7.mlp.gate_proj.weight": "pytorch_model-00001-of-00003.bin",
|
||||
"model.layers.7.mlp.up_proj.weight": "pytorch_model-00001-of-00003.bin",
|
||||
"model.layers.7.post_attention_layernorm.weight": "pytorch_model-00001-of-00003.bin",
|
||||
"model.layers.7.self_attn.W_pack.weight": "pytorch_model-00001-of-00003.bin",
|
||||
"model.layers.7.self_attn.o_proj.weight": "pytorch_model-00001-of-00003.bin",
|
||||
"model.layers.8.input_layernorm.weight": "pytorch_model-00001-of-00003.bin",
|
||||
"model.layers.8.mlp.down_proj.weight": "pytorch_model-00001-of-00003.bin",
|
||||
"model.layers.8.mlp.gate_proj.weight": "pytorch_model-00001-of-00003.bin",
|
||||
"model.layers.8.mlp.up_proj.weight": "pytorch_model-00001-of-00003.bin",
|
||||
"model.layers.8.post_attention_layernorm.weight": "pytorch_model-00001-of-00003.bin",
|
||||
"model.layers.8.self_attn.W_pack.weight": "pytorch_model-00001-of-00003.bin",
|
||||
"model.layers.8.self_attn.o_proj.weight": "pytorch_model-00001-of-00003.bin",
|
||||
"model.layers.9.input_layernorm.weight": "pytorch_model-00001-of-00003.bin",
|
||||
"model.layers.9.mlp.down_proj.weight": "pytorch_model-00001-of-00003.bin",
|
||||
"model.layers.9.mlp.gate_proj.weight": "pytorch_model-00001-of-00003.bin",
|
||||
"model.layers.9.mlp.up_proj.weight": "pytorch_model-00001-of-00003.bin",
|
||||
"model.layers.9.post_attention_layernorm.weight": "pytorch_model-00001-of-00003.bin",
|
||||
"model.layers.9.self_attn.W_pack.weight": "pytorch_model-00001-of-00003.bin",
|
||||
"model.layers.9.self_attn.o_proj.weight": "pytorch_model-00001-of-00003.bin",
|
||||
"model.norm.weight": "pytorch_model-00003-of-00003.bin"
|
||||
}
|
||||
}
|
File diff suppressed because one or more lines are too long
|
@ -0,0 +1,6 @@
|
|||
accelerate
|
||||
colorama
|
||||
cpm_kernels
|
||||
sentencepiece
|
||||
streamlit
|
||||
transformers_stream_generator
|
|
@ -0,0 +1,30 @@
|
|||
{
|
||||
"bos_token": {
|
||||
"content": "<s>",
|
||||
"lstrip": false,
|
||||
"normalized": true,
|
||||
"rstrip": false,
|
||||
"single_word": true
|
||||
},
|
||||
"eos_token": {
|
||||
"content": "</s>",
|
||||
"lstrip": false,
|
||||
"normalized": true,
|
||||
"rstrip": false,
|
||||
"single_word": true
|
||||
},
|
||||
"unk_token": {
|
||||
"content": "<unk>",
|
||||
"lstrip": false,
|
||||
"normalized": true,
|
||||
"rstrip": false,
|
||||
"single_word": true
|
||||
},
|
||||
"pad_token": {
|
||||
"content": "<unk>",
|
||||
"lstrip": false,
|
||||
"normalized": true,
|
||||
"rstrip": false,
|
||||
"single_word": true
|
||||
}
|
||||
}
|
|
@ -0,0 +1,232 @@
|
|||
# Copyright (c) 2023, Baichuan Intelligent Technology. All rights reserved.
|
||||
|
||||
import os
|
||||
from shutil import copyfile
|
||||
from typing import Any, Dict, List, Optional, Tuple
|
||||
|
||||
import sentencepiece as spm
|
||||
from transformers.tokenization_utils import AddedToken, PreTrainedTokenizer
|
||||
from transformers.utils import logging
|
||||
|
||||
|
||||
logger = logging.get_logger(__name__)
|
||||
|
||||
VOCAB_FILES_NAMES = {"vocab_file": "tokenizer.model"}
|
||||
|
||||
PRETRAINED_VOCAB_FILES_MAP = {
|
||||
"vocab_file": {},
|
||||
"tokenizer_file": {},
|
||||
}
|
||||
PRETRAINED_POSITIONAL_EMBEDDINGS_SIZES = {}
|
||||
|
||||
|
||||
class BaichuanTokenizer(PreTrainedTokenizer):
|
||||
"""
|
||||
Construct a Baichuan tokenizer. Based on byte-level Byte-Pair-Encoding.
|
||||
|
||||
Args:
|
||||
vocab_file (`str`):
|
||||
Path to the vocabulary file.
|
||||
"""
|
||||
|
||||
vocab_files_names = VOCAB_FILES_NAMES
|
||||
pretrained_vocab_files_map = PRETRAINED_VOCAB_FILES_MAP
|
||||
max_model_input_sizes = PRETRAINED_POSITIONAL_EMBEDDINGS_SIZES
|
||||
model_input_names = ["input_ids", "attention_mask"]
|
||||
|
||||
def __init__(
|
||||
self,
|
||||
vocab_file,
|
||||
unk_token="<unk>",
|
||||
bos_token="<s>",
|
||||
eos_token="</s>",
|
||||
pad_token=None,
|
||||
sp_model_kwargs: Optional[Dict[str, Any]] = None,
|
||||
add_bos_token=True,
|
||||
add_eos_token=False,
|
||||
clean_up_tokenization_spaces=False,
|
||||
**kwargs,
|
||||
):
|
||||
self.sp_model_kwargs = {} if sp_model_kwargs is None else sp_model_kwargs
|
||||
bos_token = AddedToken(bos_token, lstrip=False, rstrip=False) if isinstance(bos_token, str) else bos_token
|
||||
eos_token = AddedToken(eos_token, lstrip=False, rstrip=False) if isinstance(eos_token, str) else eos_token
|
||||
unk_token = AddedToken(unk_token, lstrip=False, rstrip=False) if isinstance(unk_token, str) else unk_token
|
||||
pad_token = AddedToken(pad_token, lstrip=False, rstrip=False) if isinstance(pad_token, str) else pad_token
|
||||
super().__init__(
|
||||
bos_token=bos_token,
|
||||
eos_token=eos_token,
|
||||
unk_token=unk_token,
|
||||
pad_token=pad_token,
|
||||
add_bos_token=add_bos_token,
|
||||
add_eos_token=add_eos_token,
|
||||
sp_model_kwargs=self.sp_model_kwargs,
|
||||
clean_up_tokenization_spaces=clean_up_tokenization_spaces,
|
||||
**kwargs,
|
||||
)
|
||||
self.vocab_file = vocab_file
|
||||
self.add_bos_token = add_bos_token
|
||||
self.add_eos_token = add_eos_token
|
||||
self.sp_model = spm.SentencePieceProcessor(**self.sp_model_kwargs)
|
||||
self.sp_model.Load(vocab_file)
|
||||
|
||||
def __getstate__(self):
|
||||
state = self.__dict__.copy()
|
||||
state["sp_model"] = None
|
||||
return state
|
||||
|
||||
def __setstate__(self, d):
|
||||
self.__dict__ = d
|
||||
self.sp_model = spm.SentencePieceProcessor(**self.sp_model_kwargs)
|
||||
self.sp_model.Load(self.vocab_file)
|
||||
|
||||
@property
|
||||
def vocab_size(self):
|
||||
"""Returns vocab size"""
|
||||
return self.sp_model.get_piece_size()
|
||||
|
||||
def get_vocab(self):
|
||||
"""Returns vocab as a dict"""
|
||||
vocab = {self.convert_ids_to_tokens(i): i for i in range(self.vocab_size)}
|
||||
vocab.update(self.added_tokens_encoder)
|
||||
return vocab
|
||||
|
||||
def _tokenize(self, text):
|
||||
"""Returns a tokenized string."""
|
||||
return self.sp_model.encode(text, out_type=str)
|
||||
|
||||
def _convert_token_to_id(self, token):
|
||||
"""Converts a token (str) in an id using the vocab."""
|
||||
return self.sp_model.piece_to_id(token)
|
||||
|
||||
def _convert_id_to_token(self, index):
|
||||
"""Converts an index (integer) in a token (str) using the vocab."""
|
||||
token = self.sp_model.IdToPiece(index)
|
||||
return token
|
||||
|
||||
def convert_tokens_to_string(self, tokens):
|
||||
"""Converts a sequence of tokens (string) in a single string."""
|
||||
current_sub_tokens = []
|
||||
out_string = ""
|
||||
prev_is_special = False
|
||||
for i, token in enumerate(tokens):
|
||||
# make sure that special tokens are not decoded using sentencepiece model
|
||||
if token in self.all_special_tokens:
|
||||
if not prev_is_special and i != 0:
|
||||
out_string += " "
|
||||
out_string += self.sp_model.decode(current_sub_tokens) + token
|
||||
prev_is_special = True
|
||||
current_sub_tokens = []
|
||||
else:
|
||||
current_sub_tokens.append(token)
|
||||
prev_is_special = False
|
||||
out_string += self.sp_model.decode(current_sub_tokens)
|
||||
return out_string
|
||||
|
||||
def save_vocabulary(self, save_directory, filename_prefix: Optional[str] = None) -> Tuple[str]:
|
||||
"""
|
||||
Save the vocabulary and special tokens file to a directory.
|
||||
|
||||
Args:
|
||||
save_directory (`str`):
|
||||
The directory in which to save the vocabulary.
|
||||
|
||||
Returns:
|
||||
`Tuple(str)`: Paths to the files saved.
|
||||
"""
|
||||
if not os.path.isdir(save_directory):
|
||||
logger.error(f"Vocabulary path ({save_directory}) should be a directory")
|
||||
return
|
||||
out_vocab_file = os.path.join(
|
||||
save_directory, (filename_prefix + "-" if filename_prefix else "") + VOCAB_FILES_NAMES["vocab_file"]
|
||||
)
|
||||
|
||||
if os.path.abspath(self.vocab_file) != os.path.abspath(out_vocab_file) and os.path.isfile(self.vocab_file):
|
||||
copyfile(self.vocab_file, out_vocab_file)
|
||||
elif not os.path.isfile(self.vocab_file):
|
||||
with open(out_vocab_file, "wb") as fi:
|
||||
content_spiece_model = self.sp_model.serialized_model_proto()
|
||||
fi.write(content_spiece_model)
|
||||
|
||||
return (out_vocab_file,)
|
||||
|
||||
def build_inputs_with_special_tokens(self, token_ids_0, token_ids_1=None):
|
||||
bos_token_id = [self.bos_token_id] if self.add_bos_token else []
|
||||
eos_token_id = [self.eos_token_id] if self.add_eos_token else []
|
||||
|
||||
output = bos_token_id + token_ids_0 + eos_token_id
|
||||
|
||||
if token_ids_1 is not None:
|
||||
output = output + bos_token_id + token_ids_1 + eos_token_id
|
||||
|
||||
return output
|
||||
|
||||
def get_special_tokens_mask(
|
||||
self, token_ids_0: List[int], token_ids_1: Optional[List[int]] = None, already_has_special_tokens: bool = False
|
||||
) -> List[int]:
|
||||
"""
|
||||
Retrieve sequence ids from a token list that has no special tokens added. This method is called when adding
|
||||
special tokens using the tokenizer `prepare_for_model` method.
|
||||
|
||||
Args:
|
||||
token_ids_0 (`List[int]`):
|
||||
List of IDs.
|
||||
token_ids_1 (`List[int]`, *optional*):
|
||||
Optional second list of IDs for sequence pairs.
|
||||
already_has_special_tokens (`bool`, *optional*, defaults to `False`):
|
||||
Whether or not the token list is already formatted with special tokens for the model.
|
||||
|
||||
Returns:
|
||||
`List[int]`: A list of integers in the range [0, 1]: 1 for a special token, 0 for a sequence token.
|
||||
"""
|
||||
if already_has_special_tokens:
|
||||
return super().get_special_tokens_mask(
|
||||
token_ids_0=token_ids_0, token_ids_1=token_ids_1, already_has_special_tokens=True
|
||||
)
|
||||
|
||||
bos_token_id = [1] if self.add_bos_token else []
|
||||
eos_token_id = [1] if self.add_eos_token else []
|
||||
|
||||
if token_ids_1 is None:
|
||||
return bos_token_id + ([0] * len(token_ids_0)) + eos_token_id
|
||||
return (
|
||||
bos_token_id
|
||||
+ ([0] * len(token_ids_0))
|
||||
+ eos_token_id
|
||||
+ bos_token_id
|
||||
+ ([0] * len(token_ids_1))
|
||||
+ eos_token_id
|
||||
)
|
||||
|
||||
def create_token_type_ids_from_sequences(
|
||||
self, token_ids_0: List[int], token_ids_1: Optional[List[int]] = None
|
||||
) -> List[int]:
|
||||
"""
|
||||
Creates a mask from the two sequences passed to be used in a sequence-pair classification task. An ALBERT
|
||||
sequence pair mask has the following format:
|
||||
|
||||
```
|
||||
0 0 0 0 0 0 0 0 0 0 0 1 1 1 1 1 1 1 1 1
|
||||
| first sequence | second sequence |
|
||||
```
|
||||
|
||||
if token_ids_1 is None, only returns the first portion of the mask (0s).
|
||||
|
||||
Args:
|
||||
token_ids_0 (`List[int]`):
|
||||
List of ids.
|
||||
token_ids_1 (`List[int]`, *optional*):
|
||||
Optional second list of IDs for sequence pairs.
|
||||
|
||||
Returns:
|
||||
`List[int]`: List of [token type IDs](../glossary#token-type-ids) according to the given sequence(s).
|
||||
"""
|
||||
bos_token_id = [self.bos_token_id] if self.add_bos_token else []
|
||||
eos_token_id = [self.eos_token_id] if self.add_eos_token else []
|
||||
|
||||
output = [0] * len(bos_token_id + token_ids_0 + eos_token_id)
|
||||
|
||||
if token_ids_1 is not None:
|
||||
output += [1] * len(bos_token_id + token_ids_1 + eos_token_id)
|
||||
|
||||
return output
|
||||
|
Binary file not shown.
|
@ -0,0 +1,46 @@
|
|||
{
|
||||
"add_bos_token": false,
|
||||
"add_eos_token": false,
|
||||
"auto_map": {
|
||||
"AutoTokenizer": [
|
||||
"tokenization_baichuan.BaichuanTokenizer",
|
||||
null
|
||||
]
|
||||
},
|
||||
"bos_token": {
|
||||
"__type": "AddedToken",
|
||||
"content": "<s>",
|
||||
"lstrip": false,
|
||||
"normalized": true,
|
||||
"rstrip": false,
|
||||
"single_word": true
|
||||
},
|
||||
"clean_up_tokenization_spaces": false,
|
||||
"eos_token": {
|
||||
"__type": "AddedToken",
|
||||
"content": "</s>",
|
||||
"lstrip": false,
|
||||
"normalized": true,
|
||||
"rstrip": false,
|
||||
"single_word": true
|
||||
},
|
||||
"model_max_length": 4096,
|
||||
"pad_token": {
|
||||
"__type": "AddedToken",
|
||||
"content": "<unk>",
|
||||
"lstrip": false,
|
||||
"normalized": true,
|
||||
"rstrip": false,
|
||||
"single_word": true
|
||||
},
|
||||
"sp_model_kwargs": {},
|
||||
"tokenizer_class": "BaichuanTokenizer",
|
||||
"unk_token": {
|
||||
"__type": "AddedToken",
|
||||
"content": "<unk>",
|
||||
"lstrip": false,
|
||||
"normalized": true,
|
||||
"rstrip": false,
|
||||
"single_word": true
|
||||
}
|
||||
}
|
Loading…
Reference in New Issue